The downside of electric cars: peak demand
At the moment, electric cars are still a niche product, but experts expect this to change sooner rather than later. Sales figures for electric vehicles are soaring. In Q1 2018, a staggering 70% more plug-ins (electric and hybrid cars) were sold than in the same period in 2017 (according to the Electric Vehicle World Sales Database), i.e. 2% of the global vehicle sales. As governments worldwide are becoming increasingly aware of air pollution, electric vehicles will become more omnipresent. In Norway, for instance, already 1 out of 3 cars sold last year were electric zero-emission cars.
But if we all switch to electric vehicles, how do we make sure that our power grid can handle the increased pressure? After all, all these cars need to be charged.
Now the charging starts immediately after a vehicle is connected to a charging station. This often leads to peaks in demand because people’s charging behavior tends to be rather similar: at a company car park, for instance, most people arrive around 9 o’clock. After parking, all of them immediately plug in their car so all vehicles are charged more or less simultaneously.
The average duration of a realistic charging session with a so-called Level 2 charger (“slow charging”) is a little over 3 hours, but cars often remain plugged into the charging station much longer. We can use this flexibility to our advantage.
“At IDLab we are working on a coordinated smart scenario where a central system – per car park or even per city – decides when each vehicle is charged. Our first objective is to avoid peak demand by maximally spreading the charging load throughout the day (‘load flattening’), but in the long run we also want to use this technique to take into account the variable nature of renewable energy (‘load balancing’),” explains Chris Develder (associate professor at ID Lab Ghent, an imec research group at the University of Ghent).
Drivers and their habits: three types of charging behavior
The first step in the research conducted by Chris Develder and his team was an extensive analysis of the biggest available dataset on electric vehicle charging, containing information on more than 2 million charging sessions at more than 2,500 public charging stations. The data was collected by ElaadNL, the knowledge and innovation center in the field of Smart Charging infrastructure in the Netherlands, and records arrival time, departure time, duration of the charging session, and the total energy consumption.
Chris Develder: “Thanks to this analysis, we got insight into people’s typical charging behavior, important input for our self-learning system. We could distinguish three types of charging sessions. More than half (62%) were ‘park to charge’ sessions, where cars head off as soon as the battery is ready to go. In this context, there is little room for flexibility. But about 28% were ‘park near home’ sessions where drivers arrive in the late afternoon and leave their car at the charging station until the next morning (cf. night charging). The third group is made up of ‘park near work’ users who arrive in the morning and leave at the end of the work day (cf. day charging). With these last two groups, there is sufficient flexibility to spread the charging load throughout the day and/or depending on the available electricity supply.”
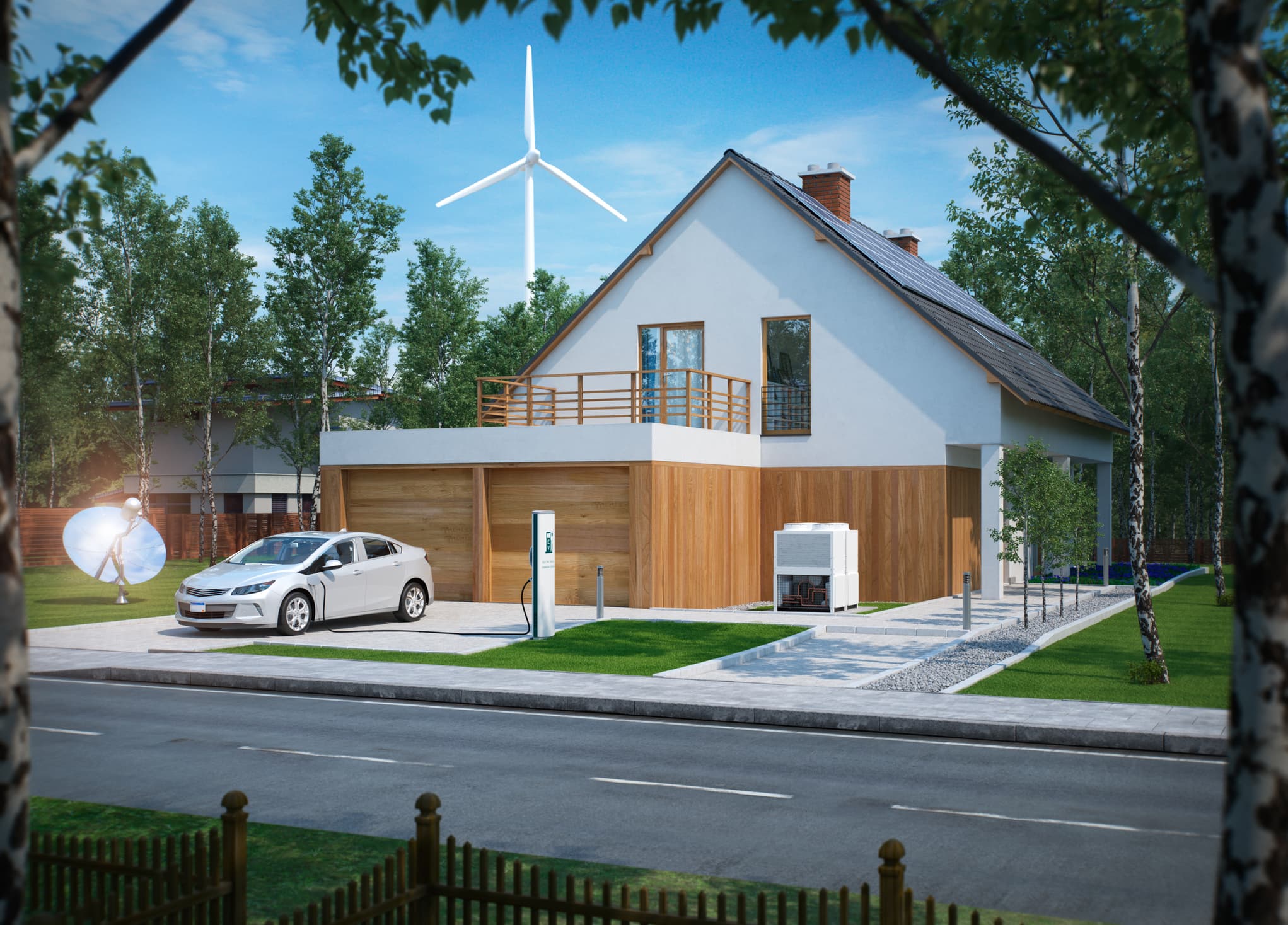
Most electric cars stay parked near a charging station much longer than they need to charge (e.g. during ‘night charging’ at home). We can use this flexibility; for instance, by charging cars only when there is excess supply of (renewable) energy and/or spreading the load evenly throughout the day to reduce peak demand.
Tackling insecurity with reinforcement learning
If you want to coordinate the charging of a large number of vehicles (ideally 100 up to even 1000 charging stations), you have to deal with a number of insecure parameters: for instance, upon arrival you can ask drivers how long they intend to stay, but you do not know how many new drivers will arrive later on. Most existing algorithms to manage our electricity consumption (i.e. demand-response algorithms) start from an established model. However, a model only works if you can control most of the parameters, which is not the case here. That is why the researchers decided on a different approach, i.e. a self-learning, model-free algorithm based on reinforcement learning.
“A self-learning algorithm based on the principle of reinforcement learning means that the system is first trained – with real-life data – to make decisions. Every decision implies a certain cost (a car was not charged in time, the energy curve was not flat enough, etc.) or reward (all cars were charged in time for departure, energy consumption was evenly spread throughout the day, etc.). Depending on the costs and rewards, the self-learning system will adapt its decision-making process, improving its performance so it becomes more and more perfect each time,” explains Chris Develder.
By observing and analyzing three months’ worth of training data by ElaadNL, the system got to know the typical charging patterns: even if it doesn’t know how many cars will arrive after 10 am on Monday morning 7 March, it can still make a pretty good guess based on data from previous Mondays and take this information into account when determining the charging schedule.
Ready for the electric car: from 10 to 1000 charging stations
After the training phase the algorithm was used to simulate the best charging schedule for a couple of test months. Its performance was compared to both the current situation (in which charging starts as soon as a car arrives) and a ‘perfect’ all-knowing scenario. The latter can only be determined in hindsight because it implies that you know how many cars will arrive throughout the day, how long they will stay and how much energy they require. In a real-life situation, this ‘perfect’ scenario is impossible because you simply don’t have this information in advance.
Chris Develder: “The performance of the algorithm depends on the number of charging stations that need to be coordinated. We’ve now tested with 10 and 50 charging stations respectively. Trained with a minimum of 3 months’ worth of training data, our solution turned out to be only 13% and 15% less efficient than the ‘perfect’ scenario. And more than 30% and 39% better than the current situation in which charging is initiated upon arrival. The more flexibility there is (i.e. the longer cars stay parked beyond the required charging time), the better our solution scores in comparison to the current situation.”
Chris Develder: “In the long run, we want to further optimize the algorithm, so we can scale to larger numbers and – as electric vehicles become more mainstream – can optimize the energy load of an entire car park or even an entire city. The advantage of reinforcement learning is that the algorithm is relatively easy to scale and generalize. We have already run a couple of simulation tests in which we trained the algorithm with data from only 10 charging stations and then used it to coordinate 100 charging stations and the results were promising.”
How demand-response algorithms can help us deal with energy shortages and overproduction
The production of renewable energy is very dependent on the weather. Simply put: if there is no wind, there is no wind energy. As renewables start to play an increasingly important role in our energy production, it also becomes more important to plan our energy consumption more carefully: not only by avoiding peak demand, but also by taking into account the variable nature of renewable energy. In other words: we have to use energy when it is being produced in excess and reduce our demand when production is low.
Demand-response algorithms can help us to do this automatically. The research on smart charging conducted by Chris Develder and his team fits within the framework of the Smile-IT project (stable multi-agent learning for networks), a strategic fundamental research project funded by VLAIO which uses artificial intelligence to optimize communication networks and electricity systems.
One part of the project focuses on how reinforcement learning can be used to develop efficient demand-response algorithms to help both small and larger players optimize their energy consumption by exploiting the flexibility in their energy usage. Other research teams specialized in energy – including the EnergyVille partners imec, VITO and KU Leuven – are also involved in this project.
EnergyVille is a collaboration between the Flemish research partners KU Leuven, VITO, imec and UHasselt that focuses on sustainable energy and intelligent energy systems. Designing a smart grid, in which the demand for energy is adapted to the energy supply instead of the other way around, is one component of the research conducted at EnergyVille.
Demand-response algorithms can do much more than only coordinate the charging of electric vehicles. They can also be used to manage our family’s energy consumption: e.g. EnergyVille researchers are currently working on a smart grid controller for household appliances. If you are away from home the whole day, there is no reason why the dishwasher, the washing machine or the robot vacuum cleaner cannot be coordinated in such a way that their energy demand matches the supply of (renewable) energy.
Want to know more?
- If you want to know more about the algorithm developed by Chris Develder and his team, you can request the full paper here.
- The research mentioned above is partly integrated into the Smile-IT project. More information can be found on their webpage.
- For more information on the smart grid controller for household appliances, take a look at the EnergyVille website.
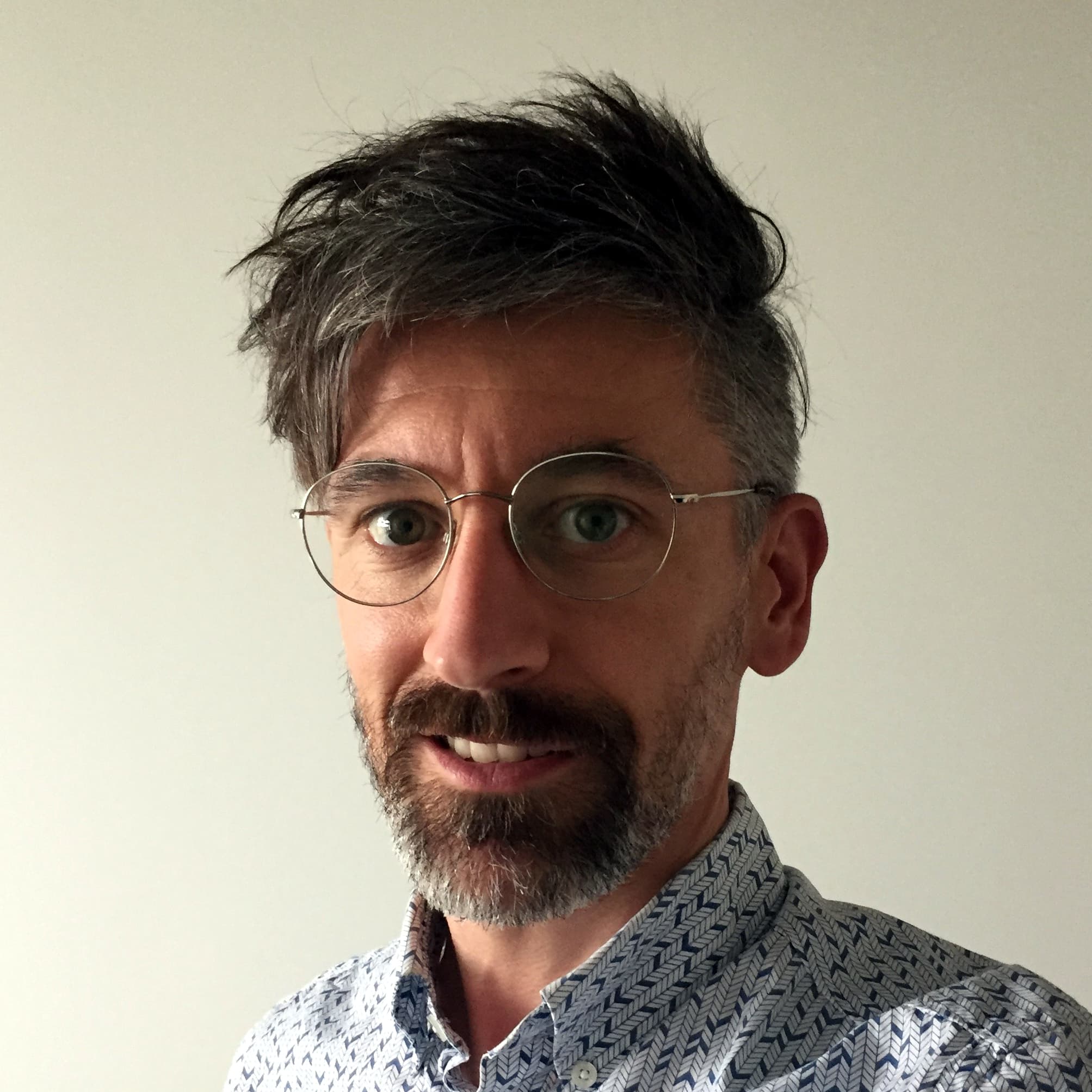
Chris Develder is associate professor at IDLab, an imec research group at Ghent University. In 1999 he received his MSc degree in computer science engineering and in 2003 he completed his PhD in electrical engineering at Ghent University. From Jan. 2004 to Aug. 2005, he worked for OPNET Technologies, on (optical) network design and planning. In Sep. 2005, he re-joined Ghent University (with an FWO scholarship from 2006 to 2012). As of February 2010, he is a fulltime professor at Ghent University. At ID Lab he leads two research teams, one on data analytics and machine learning; and another on converting text to knowledge.
Published on:
5 February 2019