Today AI is successfully used to recognize faces and traffic signs, diagnose diseases and advance remedies, predict rainfall and consumer preferences, and much more. But doing so requires powerful, energy-guzzling processors. These sit at the heart of the cloud and require sensor data to be transferred all the way from where they are generated. Which is often prohibitive. The solution? Bring AI to the sensors at the edge of the Internet of Things (IoT). To do so imec researchers – like Diederik Verkest, distinguished member of technical staff at imec – are working on a pipeline of innovative hardware that instead of hundreds of watts consume less than a watt, or even mere milliwatts.
The dominant hardware platforms involved in supervised machine learning today have top-of-line GPUs, consuming up to 200 watts. Some systems use FPGAs (field programmable gate arrays) which are on average a tad more power efficient, but which also have a corresponding lower performance. Top of the line in the performance/energy tradeoff are a number of ASICs (application specific integrated circuits), processors specifically built for deep learning. But even these will still use between 50 and 100W.
No wonder then that both machine learning and inference are now done centrally, in the cloud.
It’s simply not feasible to run a 100W dissipating chip in a mobile phone, let alone in IoT sensors that have to keep on running for longer times on much smaller energy budgets.
However, the IoT sensors are where most of the future data will be captured: wearable health sensors, vehicle-mounted radars, building-integrated stress sensors… In most cases, technical or energy constraints make it impossible to stream all that data to the cloud where the AI resides. In addition, there are also use cases where patterns should be recognized instantaneously, such as with radars that need to detect people or vehicles in the path of a self-driving vehicle. There, the time delay of a round-trip to the cloud is simply prohibitive.
So there is a great need to bring machine learning to the edge of the IoT.
For supervised learning, that doesn’t have to include the learning phase; the parameters can still be learned in the cloud. But surely inference, the smart pattern recognition, should be brought to the sensors.
But what are the energy budgets available at those nodes? Applications in vehicles, e.g., can deploy chips that use a maximum of between 10 and 30W.
But if you go to the mobile space, you’d have to do inference with 1W.
And in IoT, sensors on or in the body for example, the available budget may even be below 10mW, even going towards 1mW.
Want to know more?
- This article is based on an artcle from imec magazine from September 2018: Bringing artificial intelligence to the edge of the IoT.
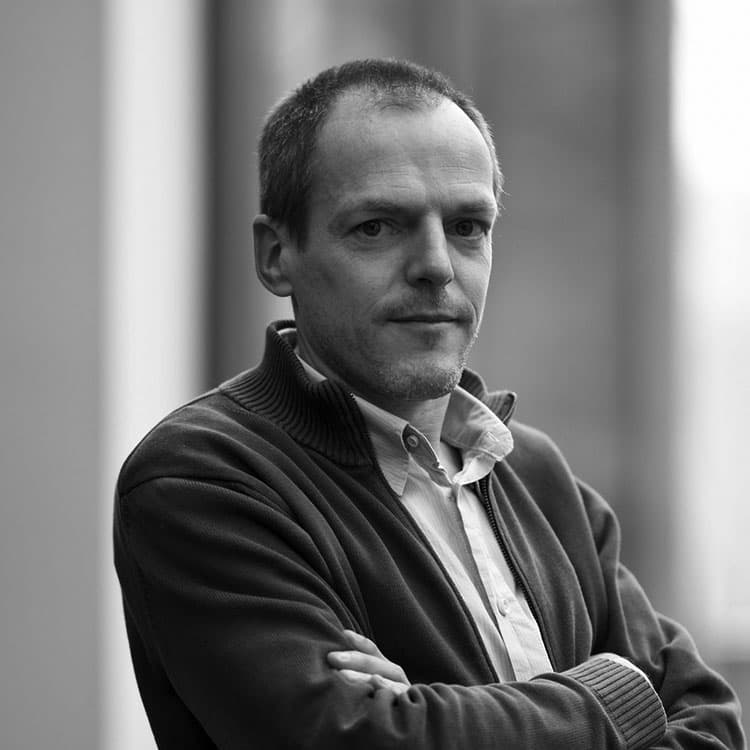
Diederik Verkest is a Distinguished Member of Technical Staff responsible for imec’s INSITE en Machine Learning programs. After earning a Ph.D. in micro-electronics engineering from the KU Leuven, Diederik joined imec in 1994, where he has been responsible amongst others for hardware/software co-design. In 2009, he started imec’s INSITE program focusing on co-optimization of design and process technology for advanced technology nodes. The program offers the fab-less design-community insights into advanced process technologies and provides a platform for foundries and fab-less to discuss directions for next generation technologies.
Published on:
13 May 2019