In April 2020, imec introduced the world’s first chip to process radar signals using a spiking recurrent neural network (SNN). Its flagship use-case? The creation of a smart, low-power multi-sensor perception system for drones that identifies obstacles in a matter of milliseconds.
Contrary to the artificial neural networks that are a key ingredient of today’s robotics perception systems, SNNs mimic the way groups of biological neurons operate – firing electrical pulses sparsely over time, and, in the case of biological sensory neurons, only when the sensory input changes. It is an approach that comes with important benefits: at the time of the announcement, imec’s SNN chip showed to consume up to a hundred times less power than traditional implementations while featuring a tenfold reduction in latency – enabling almost instantaneous decision-making.
In the following article, Ilja Ocket – program manager of neuromorphic sensing at imec – provides more insights into some of imec’s recent advances in this domain.
Optimizing and scaling up the original SNN chip
In the last year, imec has been optimizing and scaling up its original SNN chip – the details of which were recently published in ‘Frontiers in Neuroscience’ – to host a variety of (IoT and autonomous robotics) use-cases. The chip builds on an entirely event-based digital architecture, and was implemented in low-cost 40nm CMOS technology. It supports event-driven processing and relies on local on-demand oscillators and a novel delay-cell to avoid the use of a global clock. Moreover, it does not exploit separate memory blocks; instead, memory and computation are co-localized in the IC area to avoid data access and energy overheads.
Imec’s SNN ranks amongst the top performers in terms of inference accuracy
Meanwhile, research with the Dutch national research institute for mathematics and computer science (CWI), confirms that spiking neurons with adaptive thresholds can be trained to achieve top-notch inference accuracy. A comprehensive study conducted by imec and CWI aimed to benchmark SNNs using adaptive neurons against six other neural networks. To do so, eight different data sets were used – including Google’s radar (SoLi) and Google’s speech datasets. The study clearly pointed out that SNNs using neurons with adaptive thresholds can achieve a low energy consumption, but not at the expense of a decreased inference accuracy. On the contrary: for each of the major data sets used in the study, the imec SNN ranked amongst the top performers in terms of accuracy.
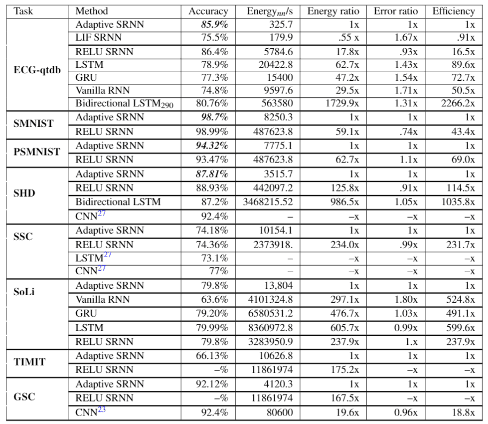
Imec’s adaptive neuron-based SNN (‘Adaptive SRNN’) was evaluated against six other neural networks – using eight different data sets including Google’s radar (SoLi) and Google’s speech datasets.
“SNN technology will find its way in a broad range of use-cases: from smart, self-learning Internet of Things (IoT) devices – such as wearables and brain-computer interface applications – to autonomous drones and robots. But each of those use-cases comes with its own set of requirements”, says Ilja Ocket. “While spiking neural networks for IoT applications should excel at operating within a very small power budget, autonomous drones demand a low-latency SNN that allows them to avoid obstacles quickly and effectively.”
“Addressing those requirements using a one-size-fits-all SNN architecture is extremely challenging. A delicate balance needs to be struck between energy consumption, latency, accuracy, cost (chip area) and scalability. For example, an SNN with the lowest possible energy consumption and latency typically results in an increased chip area – and vice versa. Finding that balance is one of imec’s SNN focus areas.”
Going forward: spiking all the way
Drones are being used in an increasing number of application domains. Still, to improve their level of autonomy and to have them operate in more challenging environments (such as bad weather conditions), their sensing capabilities require yet another boost. According to Ilja Ocket, an end-to-end spiking approach – based on fused neuromorphic radar and camera inputs – might offer a way out.
Ilja Ocket: “This obviously makes for a highly energy-efficient and super low latency system. Today, however, in order to connect such cameras to the underlying AI, one still needs to translate their feed into frames – which significantly limits the efficiency gains. That is why we are investigating how the spiking concept can be implemented end-to-end: from the cameras and sensors to the AI engine. We are actually the first ones to do so, with very promising results so far. To that end, we are still looking for companies from across the drone industry – such as OEM drone builders – to join our program and experiment with this exciting technology.”
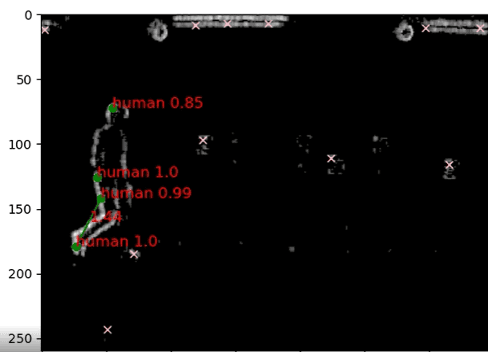
Imec’s end-to-end spiking approach at work. Local feature detection forms the first layer for a more complex semantic build-up.
Published on:
31 May 2021