Over the past two decades, data and technology have been impactful drivers behind the global healthcare and life-sciences industries. A "digital-first" mindset is a must for those who want to stay ahead of the pack. Between vision and reality, several obstacles are slowing down the uptake of technology-driven solutions. Illustrated by concrete examples, Roel Wuyts, team lead of the ExaScience Life Lab at imec, walks us through his vision on data for life sciences, the inhibitors he perceives, and how to overcome them.
In 2014, Charles Levy and David Wong coined their definition for a smart society: "[A smart society] successfully harnesses the potential of digital technology, connected devices, and the use of digital networks to improve people's lives."
Improving people's lives falls directly within the bucket of the life-sciences industry. Breaking down the Levy&Wong definition can teach us a thing or two about how digital technologies could (or: should) be harnessed within this specific sector.
Roel Wuyts: "In my opinion, the overall adoption of digital technologies and connected devices in the life-sciences industry (or the lack thereof) is a question of scaling, which in this context has an almost one-to-one relationship with data. I observe four main elements that prevent a more rapid penetration into the life-science industries. The first two are the amount of qualitative data and data accessibility. And this at the level of individuals as well as populations. The other two are the available computational resources and user adoption. For each of these inhibitors, specific technologies are under development that can help overcome them."
Smart healthcare use cases in data heaven
Why does any of this matter? What improvements can we expect to see once many of these data-related challenges are solved, taking us to the horizon of a data-enabled life-science future? Let’s look at some concrete use cases to get a glimpse of what it could look like to realize a ‘data heaven,’ driven by personalized healthcare.
And why not start with the most optimistic of them: lifestyle support? Or: the scenario where we no longer reactively manage our illness, but instead, preventively manage our health and wellness (so we rarely or never get ill). Many prevention-aware people have already adjusted their lifestyle behaviors in pursuit of a healthier quality of life. This behavior change is partly stimulated by the availability of numerous wearables for the consumer market and pushed by savvy marketing campaigns promoting wellbeing. Yet, today, these wearables largely rely on personal data alone. The next level in data-supported insights could incorporate higher-level data and statistics. Say you want to lose weight or quit smoking. A population-level comparison could help you find an approach that proved most effective for people in a similar context to your; increasing the chances that your own attempt is more likely to succeed on the first try.
While we aspire to a future without illness, there will always be a need for diagnosis, treatment, and recovery, in which data will play an increasingly important role. Presently, there is no mechanism to follow patients throughout this entire process. For example, hospitals have information on their patients’ treatments, but this is limited mainly to their specific trajectory in the hospital itself. Say you come in with a heart condition and need surgery. The hospital will meticulously manage your clinical status in preparation for, during, and after your specific heart-related treatment. But if the hospital advised you to go see an external physiotherapist or dietician, the hospital itself will not follow-up on that. And even if it did, the respective physiological or dietary data would be housed in different systems and most likely not (easily) accessible.
In future scenarios, more holistic patient management could become a reality. Creating a central ‘institution’ to house data--and giving patients access to their data-- would provide much deeper insights for patients and providers alike and immensely more control over the overall healthcare trajectory. Once centralized and data-driven healthcare management is in place, associated systems, like improved reimbursement, can follow suit. Currently, reimbursements have a direct, unique link to the type and number of interventions. This link can lead to unwanted effects like over-prescribing medicines or certain analytical tests (e.g., MRI). It can also cause indifference towards the effectiveness of diagnoses and treatments. With the availability of more precise data, other and additional valorization mechanisms could be envisaged. For example, combining a fixed financial remuneration for preventive health management with a dynamic financial scheme related to each intervention (e.g., diagnosis or treatment), and maybe even a bonus factor directly linked to their effectiveness.
With an increasing prevalence of chronic diseases, especially in Western society, a more profound follow-up becomes even more critical. Currently, drugs – be it for chronic or acute conditions - are still mostly ‘one size fits all,’ where most patients with a given condition are prescribed the same drug, even if the drug is only effective for a fraction of them. Gaining the ability to follow up on patients’ progress with (chronic) diseases more closely could substantially reduce the number of inefficient drugs prescribed. Directly related to this are the clinical trials from which these drugs result in the first place. Standard clinical trials are performed in highly controlled environments on a selection of patients that represent the average of a population. As a result, the clinical products that come out of them are also an average. To overcome the issue, pharmaceutical companies are increasingly pursuing real-world evidence for clinical trials: with data being collected in the patient’s daily environment. Using personal and contextual data in this way can eventually result in more customized drugs and treatments, with at the far side of the spectrum, personalized drugs becoming a reality for everybody.
For our final use case, we can look at genomics. A few years from now, devices will exist that – from sample to result - need four hours or less to reconstruct genetic information (e.g., DNA sequencing and analysis) digitally. Such cheap, fast, and accurate DNA sequencing will, for example, dramatically speed up the search for cures for rare diseases. And even more when combined with the already mentioned data from wearable devices, medical history, or drug intake. But it will also open new avenues to approach treatments. Imagine performing a DNA analysis every week to track the evolution of diseases such as fast-evolving cancers. That would also mean treatments could be adapted on a weekly basis, turning what are now lethal conditions into chronic ones.
Each data challenge brings a technological-development opportunity
If the benefits are that obvious, then why aren’t we there yet? What keeps us from reaching the ‘life science data heaven?’ While it’s true that data is everywhere, sufficient amounts of qualitative data are often harder to find. Let’s take video as an easy example. According to online sources, 300 minutes of video are uploaded to YouTube every minute, but algorithms fail to optimally scan and interpret its contents because the video is unlabeled. We won’t even mention the many VHS or film tapes that are literally gathering dust. The same holds true for using and generating data in the life science context. We have written diaries and logs, historical data stored in analog and digital files, the absence of measurements for large regions and populations, and many more. While artificial-intelligence solutions have become increasingly capable of also dealing with unstructured data, substantial progress is needed in the more ubiquitous availability of qualitative, labeled data. One way to achieve this is by deploying sensors, wearables, and other accurate self-calibrating devices that are networked between themselves and their environment
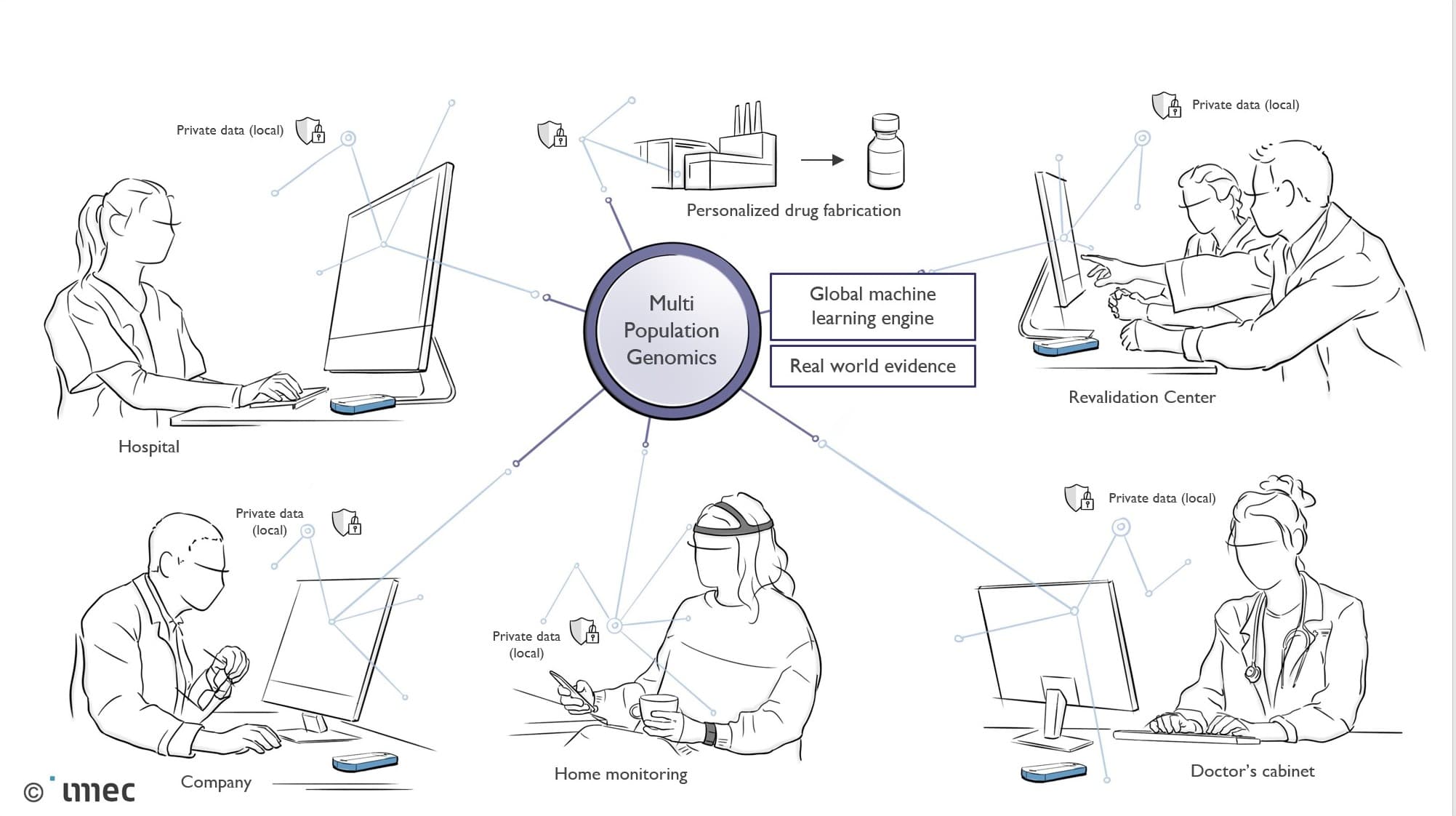
Overview of the complexity and multitude of data streams within a smart healthcare context that aspires personalized healthcare in relation to population-level analyses.
On the downside, more ubiquitous data inherently results in a need for sufficient computational resources. And computational resources are inherently power-hungry. At the moment, a lot of the complex data processing is done in the cloud, where energy and computational power are abundantly available. Yet, the fact that bitcoin processing (which is a typical example of big-data computation) globally consumes as much energy as an entire country illustrates that the attention that goes into lowering the power consumption of cloud computing is certainly not irrelevant.
On a much smaller energy scale, the quest for low power is apparent in edge computing. For various reasons (such as latency, optimal wireless communication, and privacy), edge computing requires computational intelligence as close as possible to the end-user. This applies for sensors, wearables and other devices that are not in the cloud, but literally, at ‘the edge.’
In both scenarios, cloud and edge, solutions for power-efficient computation can be found in optimized hardware and in dedicated software, with a spectrum that ranges from photonics (being implemented already) to quantum and neuromorphic computing (on the far side of exploratory research). In software development, in the most generic terms and regardless of the use case, the question is always how to make the right data available for computation at the right time. Think for a moment about DNA-analysis software (e.g., imec’s own ElPrep software). During the digital reconstruction of a genetic sequence from millions of fragments, it has to scan Gigabytes of data and make available precisely the tiny fragment that fits adjacently to the previous one. During the consecutive comparison of the genetic information with large reference databases, it must again select only those data fragments that could say something about potential anomalies or predispositions.
Unfortunately, knowing which data fragment to make available for computation at what time is only half of the story since the main question is whether that dataset is accessible in the first place. For a multitude of reasons, data can be stored in for outsiders inaccessible silos. As you could ask any pharma company, Fitbit, Apple, Facebook, Google and others, most data is proprietary, of strategic value, and hence not shared. When you request Fitbit to give you access to your personal data, they won’t provide you with your raw data, but only the information they have processed from it.
The fact that there is money to be made from data isn’t a secret, so having possession of data without (yet) knowing how to valorize it is often another reason for protective attitudes. “As long as I don’t know how to make money out of it, no one else can.” Also, legislation or mere technical difficulties can come into play because data is simply not allowed to be shared. Or the size of the data or the available bandwidth prevents it from being transferred from point A to B. One of the pathways imec and others are researching is the development of privacy-preserving machine-learning algorithms. These algorithms can access, manage, and exchange data across platforms and across owners without releasing sensitive information about the internal data, or models of those who own it. Without privacy-preserving machine-learning techniques, it will be nearly impossible to link all the necessary data sources to make personalized healthcare a reality.
Now we arrive at what is perhaps the most sensitive part: trust and user acceptance. No matter how reliable, efficient and compelling a technology; it will not be successful without winning end-user trust and convincing them of the value they can extract from your solution. Though it may not be obvious, this is also a domain where science and technology come into play. Living lab methodologies, UX design, and ethical AI are all specializations in themselves. And along the entire spectrum, from sensors and wearables, over cloud and edge computing, to data brokerage, ethics and living labs, imec deploys activities and aims to be amongst the state-of-the-art. Roel Wuyts: “Imec actively contributes to reducing or eliminating the inhibiting factors within each of these data-related contexts. Our ambition is to develop a universal platform for population-scale personalized healthcare, spanning from semiconductor technology over wearable devices to software. And holding similar value for the global healthcare industry regarding what our cleanroom facilities, research activities and partnerships mean for the semiconductor industry.
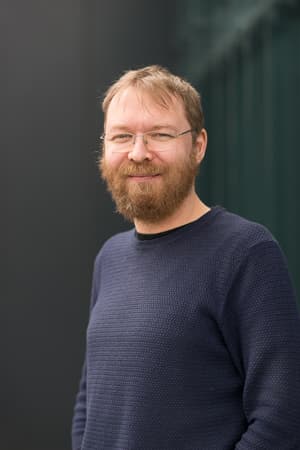
At imec Roel Wuyts leads the ExaScience Life Lab, a lab focused on providing software solutions for data-intensive high-performance computing problems, primarily in (but not limited to) the life sciences domain. He is also a part-time professor in the Distrinet group at the KULeuven. His academic achievements include published papers in IEEE Software or the Journal of Systems and Software, TOPLAS, ECOOP, OOPSLA or AOSD. He still has a special place in his heart for dynamic programming languages and was the organizer of the first Dynamic Language Symposium (DLS), co-located with OOPSLA'05.
Published on:
15 October 2020